How AI decision support systems save time and money
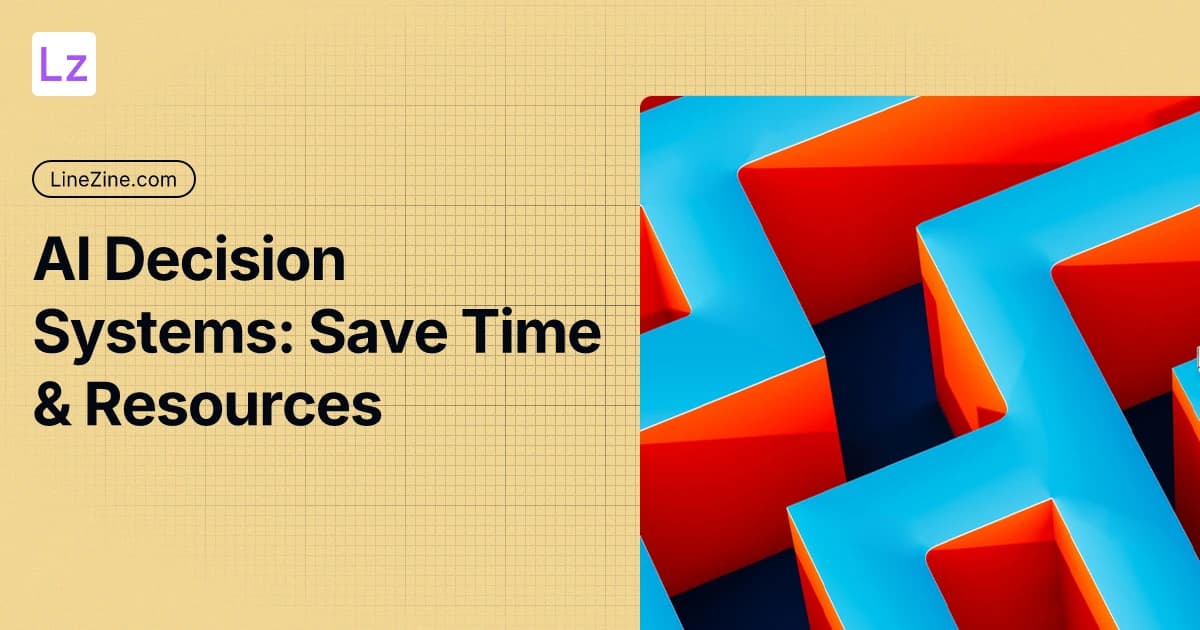
Making good choices can feel overwhelming when you're dealing with tons of information. That's where AI decision support systems come in handy. These smart tools help sort through data and give insights that lead to better decisions.
We've watched AI change how organizations make choices, turning complex numbers into useful guidance. From hospitals to banks to factories, these strategies make work faster and get better results. Let's look at what these systems can do, how they help, and what's coming next for businesses that use them.
What are AI decision support systems?
AI decision support systems blend smart algorithms with data analysis to help organizations make better choices. They process data from many sources and turn raw numbers into action plans.
These systems can:
- Predict future trends based on past data
- Process information in real-time
- Model different scenarios
- Generate automatic reports
In healthcare, they might predict how patients will respond to treatments. In finance, they assess market patterns to guide investments. In supply chains, they optimize inventory to cut costs.
The payoff is substantial. Companies using these systems make more accurate decisions, work more efficiently, and stay within regulations more easily. A retail business might use AI to decide what products to stock, when to order them, and how to price them based on customer behavior patterns.
Key parts of decision support systems
AI decision support systems rely on three main components working together to provide useful insights.
Data management
Data management is the foundation of any good decision system. It involves organizing, storing, and pulling data from various places. Good data management makes sure the information is accurate and ready to use.
Systems pull data from company databases, outside sources, and cloud services. When data management works well, it lets teams process information quickly, which means faster insights when they matter most.
Analysis models
Analysis models take raw data and find meaning in it. They use math and statistics to support predictions and run "what-if" scenarios. Common types include:
- Regression analysis
- Decision trees
- Neural networks
Each model serves a specific purpose. Some predict outcomes while others spot trends. Picking the right model depends on what kind of decision you need to make and what data you have.
User interface
How users interact with the system matters a lot. A good interface makes the system easy to use, helping people navigate through information and insights without frustration.
Effective designs include dashboards, graphs, and reports users can interact with. These features help people see complex data in ways that make sense, making the information more useful for daily decisions.
Where AI decision support systems are used
AI-powered decision systems are widely used across different industries, making data work harder for organizations.
Healthcare
In hospitals and clinics, AI systems analyze patient data to guide treatment plans. They look at health records to spot trends, helping doctors take action before problems get worse.
These systems can predict which patients might need to return to the hospital, so staff can plan resources better. AI also helps with diagnosing by finding patterns in medical images, making diagnosis faster and more accurate.
A large hospital network reported 15% fewer readmissions after implementing an AI decision support system that identified high-risk patients.
Finance
Banks and investment firms use AI to study market trends and assess risks. These systems evaluate numbers and market signals to suggest smart investment moves.
Algorithms can look at past price changes to guess future trends, helping investors make better choices. Real-time analysis spots financial risks and opportunities as they happen, improving how portfolios are managed.
Financial Application | Improvement Reported |
---|---|
Fraud detection | 37% increase in accuracy |
Portfolio management | 22% better returns |
Risk assessment | 45% faster processing |
Manufacturing
Factories use AI systems to improve supply chains and production. These systems analyze information to predict demand and manage inventory levels.
AI can predict when machines might break down, allowing for maintenance before expensive shutdowns occur. By connecting production data with market demand, manufacturers waste less and produce more efficiently.
Benefits and challenges
AI decision support systems offer important advantages but also come with some challenges. Understanding both sides helps organizations make smart choices about using them.
Advantages
- Better accuracy
AI systems use data analysis to provide precise insights, reducing human error. This accuracy helps organizations create effective plans and avoid costly mistakes.
- Greater efficiency
By automating data work, these systems speed up decision-making. This efficiency frees up people to focus on creative work instead of manual data tasks.
- Seeing the future
Advanced algorithms help predict trends before they happen. Healthcare providers can anticipate patient needs, while retailers can spot buying patterns early.
- Real-time insights
AI systems analyze data as it comes in. This immediate processing supports quick decisions when conditions change suddenly.
- Following the rules
These systems help navigate complex regulations through careful data analysis. This support keeps organizations within legal boundaries and reduces the risk of penalties.
Limitations
- Need for good data
Systems only work well with quality information. Bad data leads to poor insights, making proper data management essential.
- Setup costs
Getting started with AI systems costs money for technology and training. Organizations must weigh these costs against expected benefits.
- Over-reliance risk
Too much dependence on automated systems might weaken human decision skills. Regular training and oversight help maintain critical thinking abilities.
- Technical complexity
Advanced systems can be hard to use and understand. Users need some technical knowledge to get the most from these tools.
- Built-in bias
AI might reflect biases found in training data. This can skew analysis, making it important to regularly check how algorithms perform.
What's next for AI decision systems
The future of AI decision support systems looks promising, with several trends set to change how organizations use data.
- Custom experiences
Systems will adapt recommendations based on individual user needs. For example, a healthcare system might adjust treatment suggestions based on a patient's specific history.
- Better predictions
Improved machine learning will lead to more accurate forecasting. Companies will better predict market shifts, customer behavior, and resource needs.
- Talking to your data
Natural language processing will let users ask questions and get answers in plain language. Imagine asking, "How will changing our pricing affect quarterly sales?" and getting an immediate, data-backed response.
- Team decision-making
New tools will make it easier for teams to work together when making complex choices. Shared dashboards will let people evaluate options together in real-time.
- Responsible AI
There's growing focus on using AI ethically and transparently. Organizations will put more effort into making sure their systems are fair and trustworthy.
- Self-improving systems
Tomorrow's systems will learn continuously from new data. They'll get smarter over time without needing manual updates.
- Connected devices
Integration with internet-connected devices will feed more real-time data into decision systems. This will give organizations up-to-the-minute insights for better choices.
- Cloud access
More systems will move to the cloud, making them accessible from anywhere. Teams can work with the latest data whether they're in the office or on the road.
These advances point to a major shift in how decision support systems work. Organizations that embrace these changes will make better decisions and improve operations across their business.
Conclusion
AI decision support systems are changing how organizations make choices. By using advanced data analysis and real-time information, these systems help businesses work smarter and get better results.
These tools offer valuable insights across many industries, boosting performance in ways that weren't possible before. While there are hurdles to overcome, the advantages make these systems worth investing in.
As AI keeps advancing, decision-making will become more data-driven and intuitive. Organizations that adopt these technologies now will be better positioned for success in the future.