AI in Operations • Optimize Business Processes
Optimize your business operations with cutting-edge AI solutions. Discover how machine learning can transform supply chain management, predict maintenance needs, and automate complex workflows. Learn practical strategies for implementing AI in inventory management, quality control, and process optimization to drive efficiency and reduce costs.
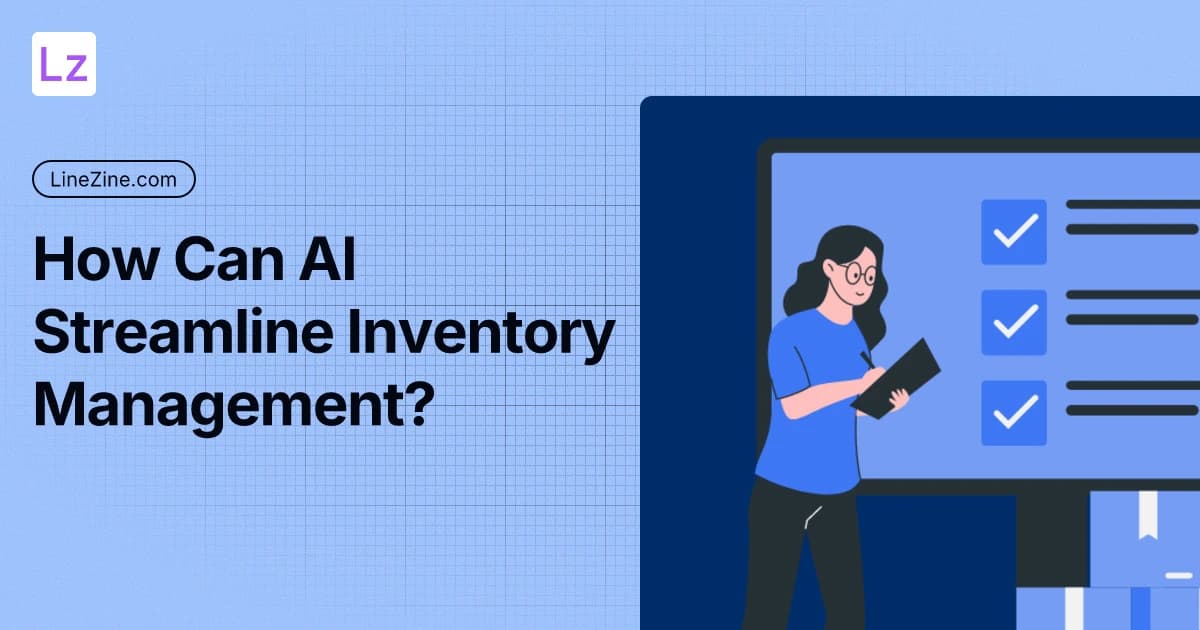
Operations
How AI forecasting transforms inventory management
Learn how AI forecasting tools predict inventory needs with amazing accuracy. Find out how businesses save money and keep customers happy with smart stock management.
July 12, 2023
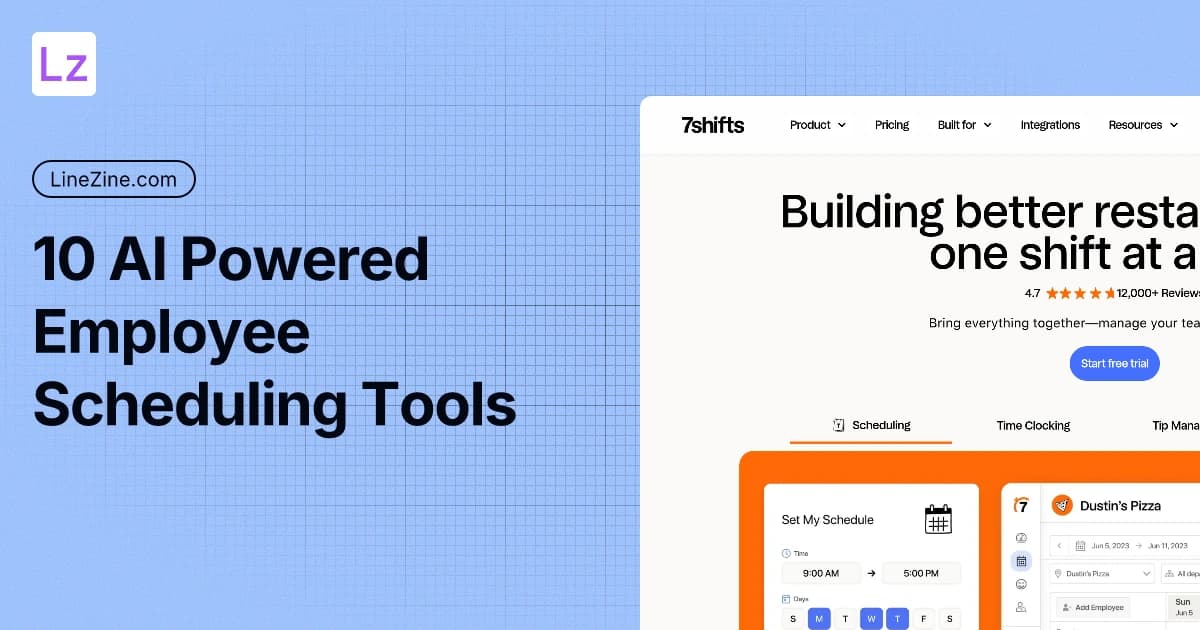
Operations
10 AI employee scheduling tools that save time and reduce costs
Discover how AI employee scheduling tools improve workforce management, boost satisfaction, and cut costs for businesses of all sizes.
March 31, 2023